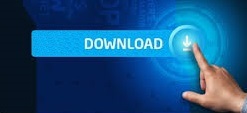
and Polak E., “ Reliability-Based Optimal Structural Design by the Decoupling Approach,” Reliability Engineering and System Safety, Vol. 73, No. 3, 2001, pp. 213–221. and Mahadevan S., “ Reliability Based Design Optimization Formulations for Component and System Reliability,” AIAA/ASME/ASCE/AHS/ASC Structures, Structural Dynamics, and Materials Conference, AIAA/ASME/AHS Adaptive Structures Conference, Vol. 48, AIAA, Reston, VA, 2008, pp. 1079–1094 also AIAA Paper 2008-1808, 2008 Google Scholar and Rais-Rohani M., “ Reliability-Based Design Optimization Within Analytical Target Cascading Framework,” AIAA/ASME/ASCE/AHS/ASC Structures, Structural Dynamics and Materials Conference, AIAA/ASME/AHS Adaptive Structures Conference, Vol. 45, AIAA, Reston, VA, 2013, pp. 795–799 also AIAA Paper 2012-1847, 2012. F., A Comparison of Some Algorithms for Reliability-Based Structure Optimization and Sensitivity Analysis, Vol. 76, Springer, Berlin, 2015, pp. 443–451. M., “ Reliability-Based Design Optimization Using Kriging Surrogates and Subset Simulation,” Structural and Multidisciplinary Optimization, Vol. 44, No. 5, 2011, pp. 673–690. and Park Y., “ A New Study on Reliability-Based Design Optimization,” Journal of Mechanical Design, Vol. 121, No. 4, 1999, pp. 557–564. and Kapania R., “ New Approach for System Reliability-Based Design Optimization,” AIAA Journal, Vol. 129, No. 44, 2012, pp. 1087–1096. D., “ Reliability-Based Optimization in Structural Engineering,” Structural Safety, Vol. 15, No. 3, 1994, pp. 169–196. and Mahadevan S., “ A Direct Decoupling Approach for Efficient Reliability Based Design Optimization,” Structural and Multidisciplinary Optimization, Vol. 31, No. 3, 2006, pp. 190–200. and Wei D., “ Design Sensitivity and Reliability-Based Structural Optimization by Univariate Decomposition,” Structural and Multidisciplinary Optimization, Vol. 35, No. 3, 2008, pp. 245–261. D., “ Efficient Algorithm for Second-Order Reliability Analysis,” Journal of Engineering Mechanics, Vol. 117, No. 12, 1991, pp. 2904–2923. and Olsson M., “ Reliability Based Design Optimization Using a Single Constraint Approximation Point,” Journal of Mechanical Design, Vol. 133, No. 3, 2011, Paper 031006. H., “ A New Study on Reliability-Based Design Optimization,” Journal of Mechanical Design, Vol. 121, No. 4, 1999, pp. 557–564. and Yun W., “ Aircraft Icing Severity Analysis Considering Three Uncertainty Types,” AIAA Journal, Vol. 57, No. 6, 2019, pp. 1–9. H., “ Reliability Based Design Optimization with Approximate Failure Probability Function in Partitioned Design Space,” Reliability Engineering and System Safety, Vol. 167, Nov. 2017, pp. 602–611. Meanwhile, the design optimization of a stiffened rib of the wing edge in a certain aircraft also verifies its good engineering applicability. The computation capability of the proposed method is demonstrated by several analytical RBDO problems. Because the sparse PCEs are built in an augmented space, it is made numerically affordable to solve the RBDO problem with no extra computational cost. Two goals can be achieved using this criterion that is, most of the selected training points are selected from the desired regions to approximate limit state surfaces, and these points tend to be far away from the existing points in the current design to avoid the clustering problem. In the meanwhile, a new active learning function is proposed to adaptively select new training points. Because classic sparse regression methods cannot provide the surrogate error measure that can be employed to improve the sampling performance for failure probability estimation in RBDO, Bayesian compressed sensing with state-of-the-art performance is employed to build sparse PCE in the paper. The aim of the present paper is to develop a strategy for solving reliability-based design optimization (RBDO) problems by sparse polynomial chaos expansion (PCE).
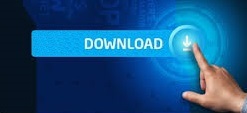